Employing Large Language Models (LLMs) for several different tasks, spanning from daily life errands, or simply entertainment, to advanced applications and experimental use within any scientific domain, has been on the hype for quite a while now. LLMs have been under the microscope of the scientific community, not only regarding IT-related subjects, but after recognising a potential worthy of investigation, plenty of multidisciplinary research has been ongoing, aiming for off-the-beaten-track scientific discovery.
Following the same rationale, for someone working with open and linked data and aiming to establish connections and semantic relations, LLMs become appealing; one cannot help but think: How can then these models facilitate the process of linking knowledge?
Multidisciplinary research and data
Especially when thinking of multidisciplinarity, scientific research nowadays is ever surpassing the narrow limits of one knowledge domain, forming a vastness of combinations of domains coupled (social science and computer science, computer science and environmental science, environmental science and physics, psychology and computer science, architecture and environmental science, and the list is long). A level of mutual understanding -a consensus- among the notions, tools, methods, and techniques native to each domain can facilitate a deeper comprehension of machine-readable and processable data, allowing for machines (algorithms) to identify links and existing connections among seemingly not-so-relevant concepts, just like an experienced human mind with multidisciplinary knowledge stimulus can spot conceptual correlations.
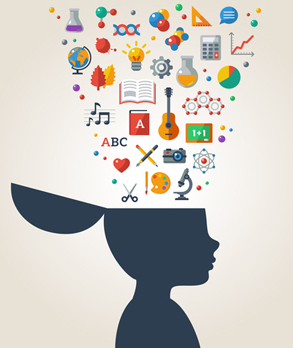
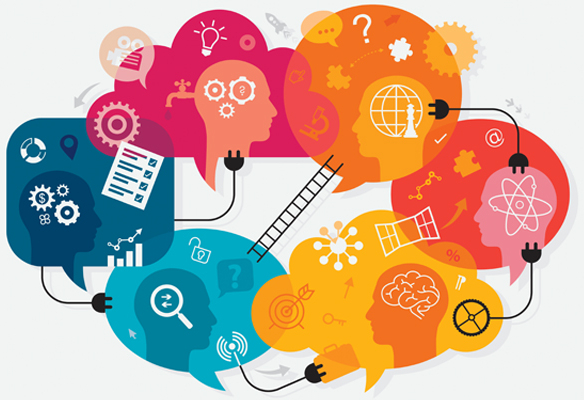
At the same time, research is ever relying on data, while data is becoming the new oil of our times. Algorithms (ML, LLMs, GenAI) also need data, a lot of data, and more so, data of good quality. But how can data become easier to find (discoverable) and have high semantic value?
The role of ontologies and data models in facilitating search capabilities within large collections of data is critical; the integration and analysis of diverse data sources becomes feasible as ontologies frame the data conceptually and provide a common understanding of terms and their relationships- the lack of ontological and conceptual support entailing the opposite effect. Along with documents and data lost in the vastness of available yet disparate data sources, numerous scientific papers and published research remain undiscovered due to poor linking to their respective scientific domain and investigation method(s) described in them.
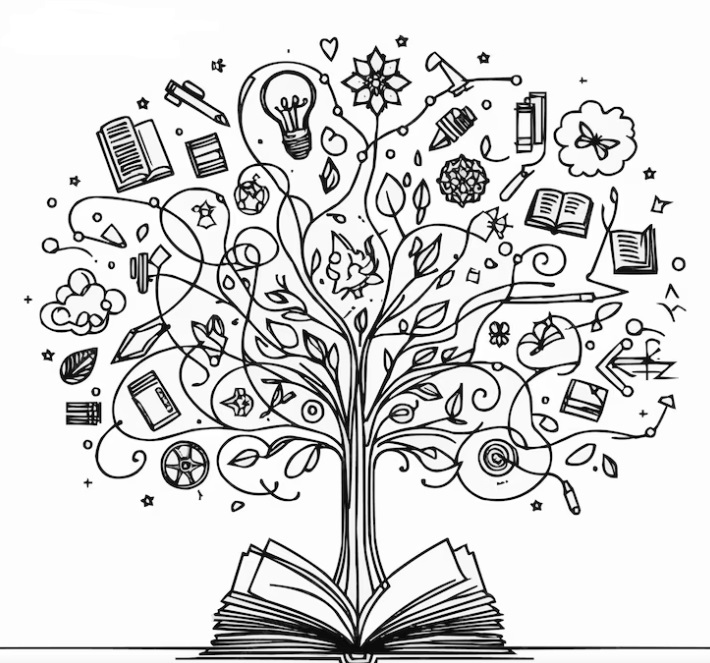
For instance, if a researcher tries to fetch “Concepts/Methods in X Discipline”, there is no structured, uniform, or predictable way to retrieve content. Research content from scientific articles can often be retrieved by keyword searches of the respective scientific domain one wishes to explore, however, the case is not that trivial when the domain is not explicitly mentioned in the article’s metadata, when the research falls into multidisciplinarity, or when it uses shared scientific methodological tools which belong to more than one domain, making it difficult to retrieve relevant results.
Knowledge as a graph
In a practical example, let us consider Wikidata, one of the most important central repositories- a knowledge base.
Why is it not feasible to easily retrieve a comprehensive list of methods across scientific disciplines with a simple SPARQL query?
As mentioned before, knowledge representation is key, and so is the structure (data model) the data is following. To make it simple, let us imagine a structure, representing all Wikidata codes (data existing on Wikidata) which refer to scientific investigation tools, methods, and terms. The graph, in this case, expresses the hierarchical and conceptual relationships among those codes.
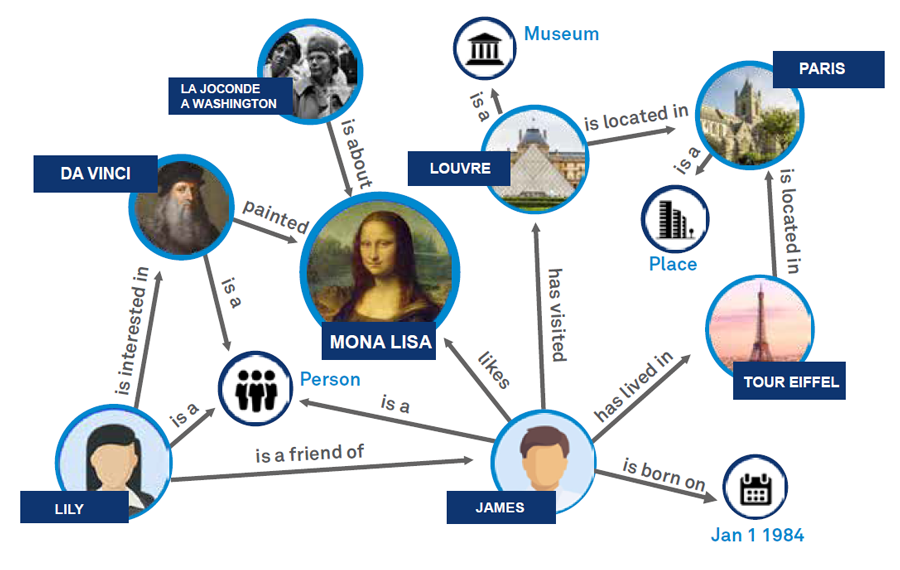
Should one be able to enhance the structure of relationships between the different notions, methods, and tools, capturing the right level of specificity but also the (at least one of) conceptually suitable places where a notion belongs, they immediately make the notion in-word discoverable by a query including any of the relatable concepts, even if those belong to different disciplines, as long as the relationships of the data are represented in the “tree of knowledge” (in other words: a knowledge graph).
LLMs at the service of knowledge representation
During my recent professional secondment at the Greek Open Technologies Alliance (GFOSS), we experimented with employing ChatGPT and GPT-4 on the Wikidata paradigm, to see how they can facilitate the process of identifying and enriching data relationships to make otherwise-impossible-to-find data discoverable.
The initial findings of our study on Wikidata codes revealed several issues regarding discoverability and showing limited semantic search capabilities to retrieve multidisciplinary scientific investigation methods and tooling for a couple of chosen disciplines (starting with the examples of Psychology, Cultural Heritage, and Neuroscience and then expanding to more).
If LLMs can be utilised to identify “orphan” categories (currently not belonging to a sound hierarchical structure) and, by benchmarking against known and standardised ontologies for each scientific domain, can propose where new data categories could be placed in the “tree of knowledge, many new data capabilities are unlocked. Then, taking the best of human and machine understanding, we are entering a new, technologically-enabled era for semantic interoperability and the discoverability of data.
Why? Because with the rise of disruptive technologies and the increase of multidisciplinary approaches for every IT-related subject (but not limited to), interlinked knowledge sources become pivotal for the promotion of social development, research, and innovation.
Author:
Maria Ioanna Maratsi, University of the Aegean